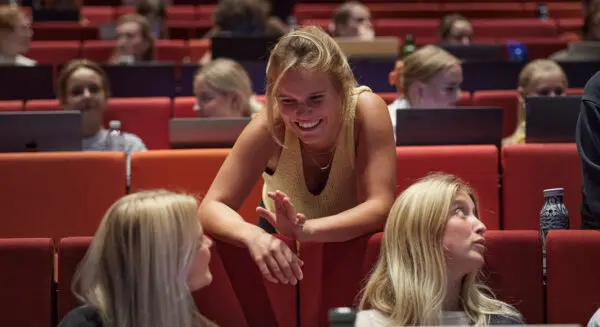
Copenhagen, Denmark
Forensic Genetics and Massively Parallel Sequencing
When:
11 August - 22 August 2025
Credits:
5 EC
Read more
Life Sciences
When:
15 August - 19 August 2022
School:
Institution:
Radboud University
City:
Country:
Language:
English
Credits:
2 EC
Fee:
550 EUR
Do you have large-scale neuroscience datasets and lots of ideas, but need a better understanding of how to work with your data? Then this course is for you! You will learn key concepts in multichannel neuroscience analyses, including dimension-reduction, source separation, and least-squares modeling, and how to implement them in Matlab.
Dr. Michael X Cohen, Associate professor Donders Institute for Brain, Cognition and Behaviour Radboud University Medical Center
-PhD
-Post-doc
-Professional
This course is designed for PhD students, postdocs, and senior researchers who have experience with data analysis and want a deeper understanding of advanced data analysis methods. Some experience with Matlab is necessary. Masters students are welcomed if they have had some experience with neuroscience data analysis. The course focuses on analog electrophysiology signals (LFP/EEG/MEG), but the methods are applicable to imaging (fMRI or calcium/wide-field imaging) as well.
After this course you are able to:
1. Understand the key concepts in linear algebra including matrix multiplication, inverse, and projections, as well as know geometric and algebraic ways of representing data and analyses.
2. Implement the least-squares algorithm to estimate general linear model.
3. Understand eigendecomposition and its use in dimensionality reduction and source separation.
4. Simulate multivariate data to evaluate analysis methods and model overfitting.
Fee
550 EUR, The fee includes the registration fees, course materials, access to library and IT facilities, coffee/tea, lunch, and a number of social activities.
When:
15 August - 19 August 2022
School:
Institution:
Radboud University
Language:
English
Credits:
2 EC
Copenhagen, Denmark
When:
11 August - 22 August 2025
Credits:
5 EC
Read more
Pisa, Italy
When:
21 July - 24 July 2025
Credits:
3 EC
Read more
Coventry, United Kingdom
When:
15 July - 25 July 2025
Credits:
0 EC
Read more