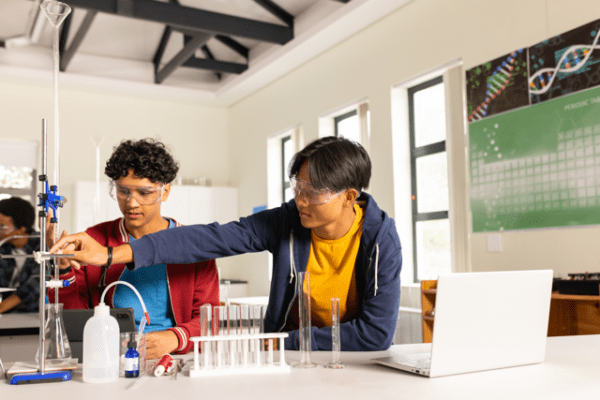
Antwerp, Belgium
Inter- and Transdisciplinary Research
When:
18 August - 22 August 2025
Credits:
3 EC
Read more
Social Sciences
When:
18 August - 22 August 2025
School:
Summer School in Social Sciences Methods
Institution:
UniversitΓ della Svizzera italiana
City:
Country:
Language:
English
Credits:
0 EC
Fee:
700 CHF
Workshop Contents and Objectives
In this course, you will learn how to create groups from data. For example, you might want to detect different types of web users based on a set of variables that contain information on aspects of online activities (e.g., content preferences, time spent online, etc.). Or you try to understand vaccination hesitancy by identifying groups of people with similar sets of concerns. In these kinds of scenarios, we often face datasets with many observations and large numbers of potentially relevant variables. It would be impossible to find groups of similar cases by just browsing the data or skimming tables.
Discovering groups in your data can be achieved by performing cluster analysis or latent class analysis. These techniques allow for a fruitful description of the phenomenon of interest and enable follow-up analyses, for example, on how group membership (e.g., type of web users) is associated with other variables (e.g., gender, SES, life satisfaction, personality).
Cluster analysis can be described as a bottom-up approach, where various algorithms are deployed to find similar cases (e.g., persons, organisations, schools, countries) in your data. Similar cases will be grouped to create a given number of maximally different clusters. Latent class analysis, in contrast, can be seen to use a top-down approach where we assume a probabilistic model to explain group membership. Latent class analysis works with the actual distribution of your data using a statistical model. You can include covariates, and the procedure will provide goodness of fit measures, which can be used to compare different solutions.
This course contains an introduction to both cluster analysis and latent class analysis. We will spend three days on cluster analysis (hierarchical cluster analysis, non-hierarchical clustering, k-means, fuzzy clustering, all for continuous, categorical, and mixed variables) and two on latent class analysis (also covering latent profile analysis and longitudinal applications).
You will work on data provided by the course instructor. We encourage you to bring your data if possible. Upon completion of this course, you will have a good understanding of cluster analysis and latent class analysis, and their differences, advantages, and disadvantages. You will know how to use these techniques with your data
Workshop design
Fifty-fifty mix of interactive lectures and exercise sessions. Exercises can be done in groups. Daily feedback and possibilities for individual consulting.
Detailed lecture plan (daily schedule)
Day 1
Introduction to Cluster Analysis, Hierarchical Cluster Analysis
Day 2
Non-Hierarchical Clustering
Day 3
Fuzzy Clustering and Advanced Applications
Day 4
Introduction to Latent Class Analysis, Modelling Covariates
Day 5
Advanced Latent Class Analysis (Constrained, Multigroup, Longitudinal), Latent Profile Analysis
Class materials
Slides
Exercise scripts, annotated solutions, additional illustrative scripts (all in R)
Set of exemplary papers
Selected readings by topic
**The Summer School cannot grant credits. We only deliver a Certificate of Participation, i.e. we certify your attendance.**
If you consider using Summer School workshops to obtain credits (ECTS), you will have to investigate at your home institution (contact the person/institute responsible for your degree) to find out whether they recognise the Summer School, how many credits can be earned from a workshop/course with roughly 35 hours of teaching, no graded work, and no exams.
Make sure to investigate this matter before registering if this is important to you.
Robin Samuel is an Associate Professor at the University of Luxembourg (Department of Social Sciences) and Head of the Centre for Childhood and Youth Research.
graduate students, doctoral researchers, early career researchers, experienced researchers
Prerequisites
Participants should be familiar with univariate and bivariate statistics. If you have never been exposed to bivariate correlation and chi-square (e.g., in the context of crosstabs, also known as contingency tables) this is probably not the course for you. Ideally, you will have some knowledge of OLS and logistic regression as well.
We will use the software R. R allows running cluster analyses and finite mixture models (e.g., latent class analysis and latent profile analysis). While familiarity with R would be useful, this is not strictly necessary if you have some knowledge of working with other statistical software packages using syntax (e.g., Stata or SPSS) and are willing to learn. You must be able to perform basic data management tasks in R or another software (e.g., recoding of variables, missing values, etc.).
Here are some helpful materials for those who are new to R or feel they would benefit from a refresher: https://stats.idre.ucla.edu/r/
Fee
700 CHF, Reduced fee: 700 Swiss Francs per weekly workshop for students (requires proof of student status). To qualify for the reduced fee, you are required to send a copy of an official document that certifies your current student status or a letter from your supervisor stating your actual position as a doctoral or postdoctoral researcher. Send this letter/document by e-mail to methodssummerschool@usi.ch.
Fee
1100 CHF, Normal fee: 1100 Swiss Francs per weekly workshop for all others.
When:
18 August - 22 August 2025
School:
Summer School in Social Sciences Methods
Institution:
UniversitΓ della Svizzera italiana
Language:
English
Credits:
0 EC
Antwerp, Belgium
When:
18 August - 22 August 2025
Credits:
3 EC
Read more
Maastricht, Netherlands
When:
14 July - 18 July 2025
Credits:
2 EC
Read more
Brno, Czechia
When:
13 July - 26 July 2025
Credits:
4 EC
Read more