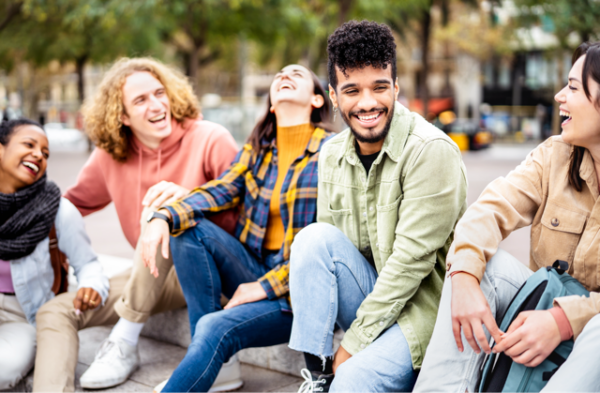
Rhodes, Greece
Summer School 2025 “Small Acts. Big Impact”
When:
18 September - 16 October 2025
Credits:
0 EC
Read more
Social Sciences
When:
03 August - 05 August 2022
School:
GESIS Summer School in Survey Methodology
Institution:
GESIS-Leibniz Institute for the Social Sciences
City:
Country:
Language:
English
Credits:
0 EC
Fee:
200 EUR
This online short course uses causal graphs (or βdirected acyclic graphsβ, DAGs) as a remarkably simple, yet general and powerful framework to describe and discuss a large set of problems that empirical social scientists need to tackle. Is my question of interest descriptive or causal? How can I communicate my assumptions effectively to others, and can I test them? How can I tell correlation from causation? How do I choose control variables for my regression models? After discussing how DAGs can be used to answer these foundational questions, the course also covers basics of causal interaction and effect heterogeneity, causal mediation, nonresponse/selection bias (and adjustments for it) and, if time permits, instrumental variables and panel data analysis from a DAG perspective.
Julian Schuessler is a post-doc at the Institute for Political Science, Aarhus University, Denmark.
Participants will find the course useful if they are:
- interested in causal questions and want to understand the assumptions associated with regression control, mediation analysis, and instrumental variables better:
- interested in non-causal questions, want to use data suffering from nonresponse, and want to understand how to use causal assumptions in this case.
Prerequisites:
Participants should be willing to learn and use formal reasoning and must have at least Bachelor-level knowledge of statistics. Basic knowledge of R is helpful.
By the end of the course participants will:
- know how to use causal graphs to visualize causal assumptions, define quantities of interest, and determine testability of assumptions via d-separation;
- know how to graphically determine the identification of causal and descriptive quantities like average causal effects, causal interaction, effect heterogeneity, natural direct and indirect effects, and population distributions from data with nonresponse;
- know under what graphical assumptions instrumental variable and panel data analysis typically operate;
- will have some basic knowledge about how all of this relates to implementation in standard statistical software.
Fee
200 EUR, Student/PhD student rate.
Fee
300 EUR, Academic/non-profit rate. The rates include the tuition fee and course materials.
When:
03 August - 05 August 2022
School:
GESIS Summer School in Survey Methodology
Institution:
GESIS-Leibniz Institute for the Social Sciences
Language:
English
Credits:
0 EC
Rhodes, Greece
When:
18 September - 16 October 2025
Credits:
0 EC
Read more
Utrecht, Netherlands
When:
11 August - 22 August 2025
Credits:
1.5 EC
Read more
Utrecht, Netherlands
When:
09 July - 11 July 2025
Credits:
1.5 EC
Read more